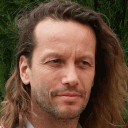

Rufin VanRullen
ERC Advanced GLoW
We are hiring!!!
- We are interested in competent and motivated post-doc and PhD candidates.
- Below are some details on the positions available in Fall 2023, but we will be continuing to hire on a rolling basis in the foreseeable future. Drop me an email (with your CV and background info) if you're interested.
Multiple postdoctoral and PhD positions available to work on an ERC-funded project exploring deep-learning implementations of the Global Workspace theory.
We are seeking highly motivated and dedicated students and post-docs to join our group in Toulouse, France. Successful candidates will have formal training in AI, Computer Science or related fields (e.g. computational neuroscience), and a keen interest for cognitive neuroscience. We aim to take inspiration from neuroscience and cognitive theories to build deep neural network models of cognition, capable of integrating or "grounding" information across sensory and linguistic modalities, and of displaying flexible cognitive behavior. We will also use advanced AI models to improve the decoding and understanding of brain activity. Details on the ideas that will be developed in the project can be found in this Opinion paper.
Our lab is part of the CerCo Institute as well as the recently created Artificial and Natural Intelligence Toulouse Institute (ANITI). Our team has many opportunities to collaborate with neuroscientists, computer scientists, linguists, roboticists etc. We have direct access to fMRI recording facilities and to in-house (GPU) computing clusters as well as research-dedicated super-computers.
Typical appointments for post-docs are for 2 years (renewable for a 3rd or 4th year), and 3-years for PhD. The beginning post-doc salary is 2,590 Euros/month (net), and can reach 3,200 Euros/month (net) for more experienced post-docs. The PhD stipend is 2,000 Euros/month (net). We expect to hire several candidates by the Fall 2023, but will continue to recruit exceptional applicants in the foreseeable future.
To apply, please send a CV and a letter explaining your research interests and motivations to: rufin.vanrullen [at] cnrs.fr
Any questions should also be directed to the same address.
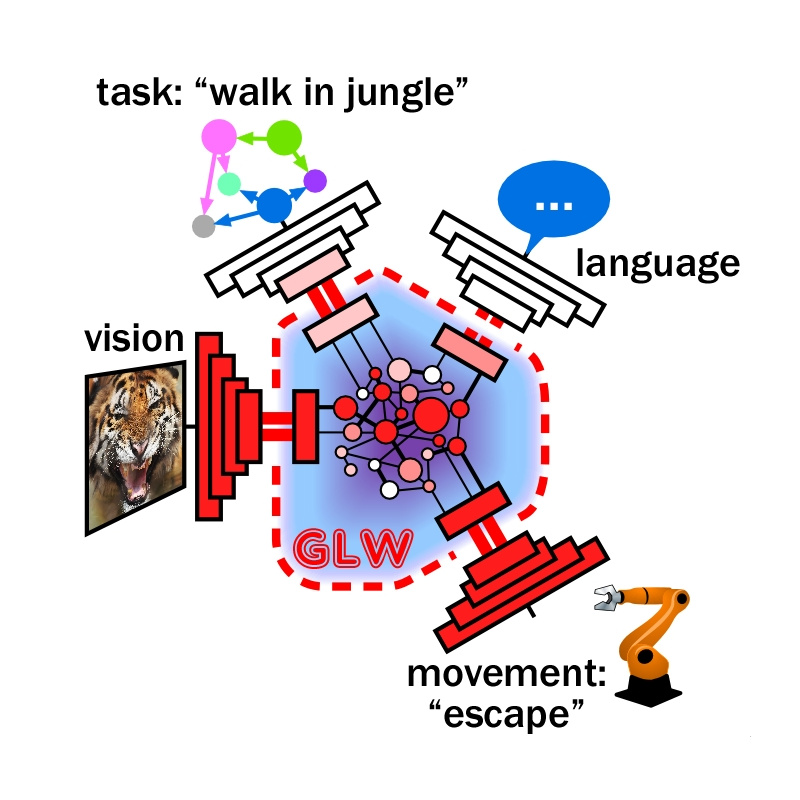
ERC Advanced GLoW
The GLobal Latent Workspace: Towards AI models of flexible cognition
Recent advances in deep learning have allowed Artificial Intelligence (AI) to reach human-level performance in many sensory, perceptual, linguistic or cognitive tasks. There is a growing need, however, for novel, brain-inspired cognitive architectures to achieve more robust and flexible cognition. The Global Workspace Theory refers to a large-scale system integrating and distributing information among networks of specialized modules to create higher-level forms of cognition and awareness. It is one of the dominant neuroscientific accounts of higher-level brain function. We argue that the time is ripe to consider explicit implementations of this theory using deep learning techniques. We propose a roadmap based on unsupervised neural translation between multiple latent spaces (neural networks trained for distinct tasks, on distinct sensory inputs and/or modalities) to create a unique, amodal global latent workspace (GLW). Sensory inputs that are broadcast in this GLW acquire meaning by connecting them to (or translating them into) the relevant semantic knowledge and representations from language, memory or decision systems: the semantic grounding property. Conversely, language and semantic representations are grounded in the sensory environment via the same broadcast/translation operation. Finally, broadcasting sensory and semantic inputs to the relevant effector domains can create motor affordances and support embodied cognition. Together, the grounding and affordance properties infuse meaning in AI processes, which can then be combined sequentially (via attentional selection) to enable flexible cognitive functions—i.e., “System-2” AI. The interdisciplinary project will directly implement the GLW framework in deep learning models of growing complexity, and evaluate their correspondence with brain networks. It will provide an explicit evaluation of the Global Workspace Theory, and push the limits of current deep learning systems towards next-generation AI.